AI Doesn’t Mean Instant Answers
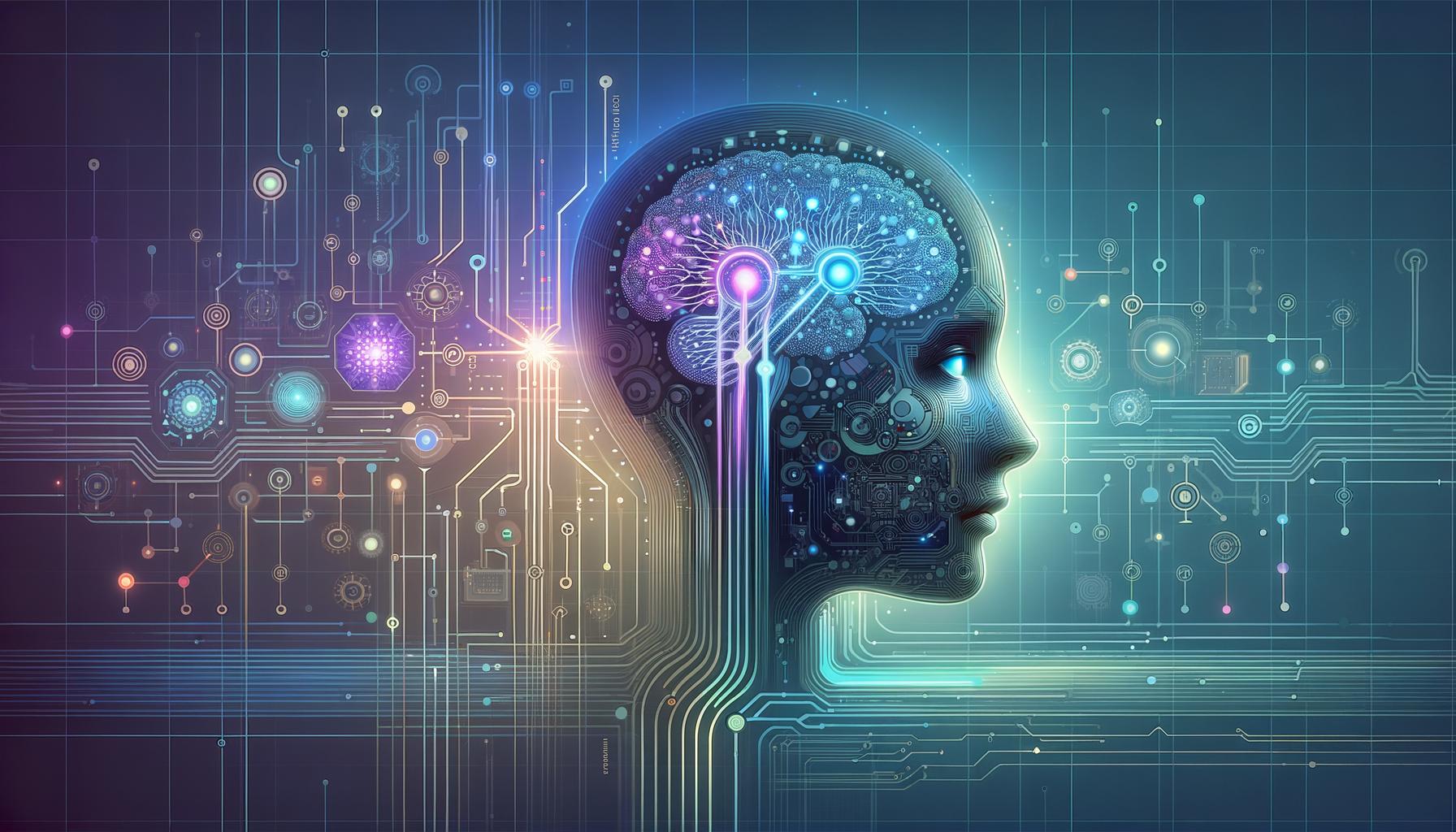
The Myth of Instant, Flawless AI Answers
Let’s get honest about something I’ve seen crop up everywhere—from boardrooms to group chats to late-night dev forums: the idea that AI is an all-knowing, flawless genie. Push a button, get a perfect answer. Who wouldn’t want that? Especially in engineering, where the pressure to solve big, hairy problems is constant. I get it. The fantasy is tempting: an expert that never sleeps, never second-guesses, and never asks for a raise.
But if you’ve spent any real time collaborating with AI on engineering work, you know it’s not that simple. Not even close.
Automation is powerful. No argument there. But here’s where this gets real: when engineers start believing AI is a substitute for their own judgment, things get risky fast. I’ve seen it firsthand—and so have others. One industry expert put it bluntly: misplaced trust in AI is already showing consequences in critical sectors. In healthcare, chatbots dispensing unchecked advice can endanger patients and privacy. In finance, a slip means sensitive data is exposed; in manufacturing, a careless output could stall production or worse; and in aviation? Even a small error can undermine safety, as shown by the real-world consequences of AI failures.
I remember reading about a major hospital network rolling out an AI diagnostic tool back in 2018—expecting instant magic. What happened? The system flagged potential issues at lightning speed but completely misread rare conditions. It took seasoned physicians double-checking its suggestions to catch the misses. That story sticks with me because it’s so familiar: new tech comes in, and everyone wants to trust it… until reality reminds us why human oversight matters, especially when the stakes are high.
So let’s call out the myth of perfect AI for what it is—and talk about what really works. This post isn’t about dismissing AI; it’s about redefining the relationship. The goal? Treat AI not as an all-knowing authority, but as a collaborative partner—one that can multiply your expertise if (and only if) you stay engaged.
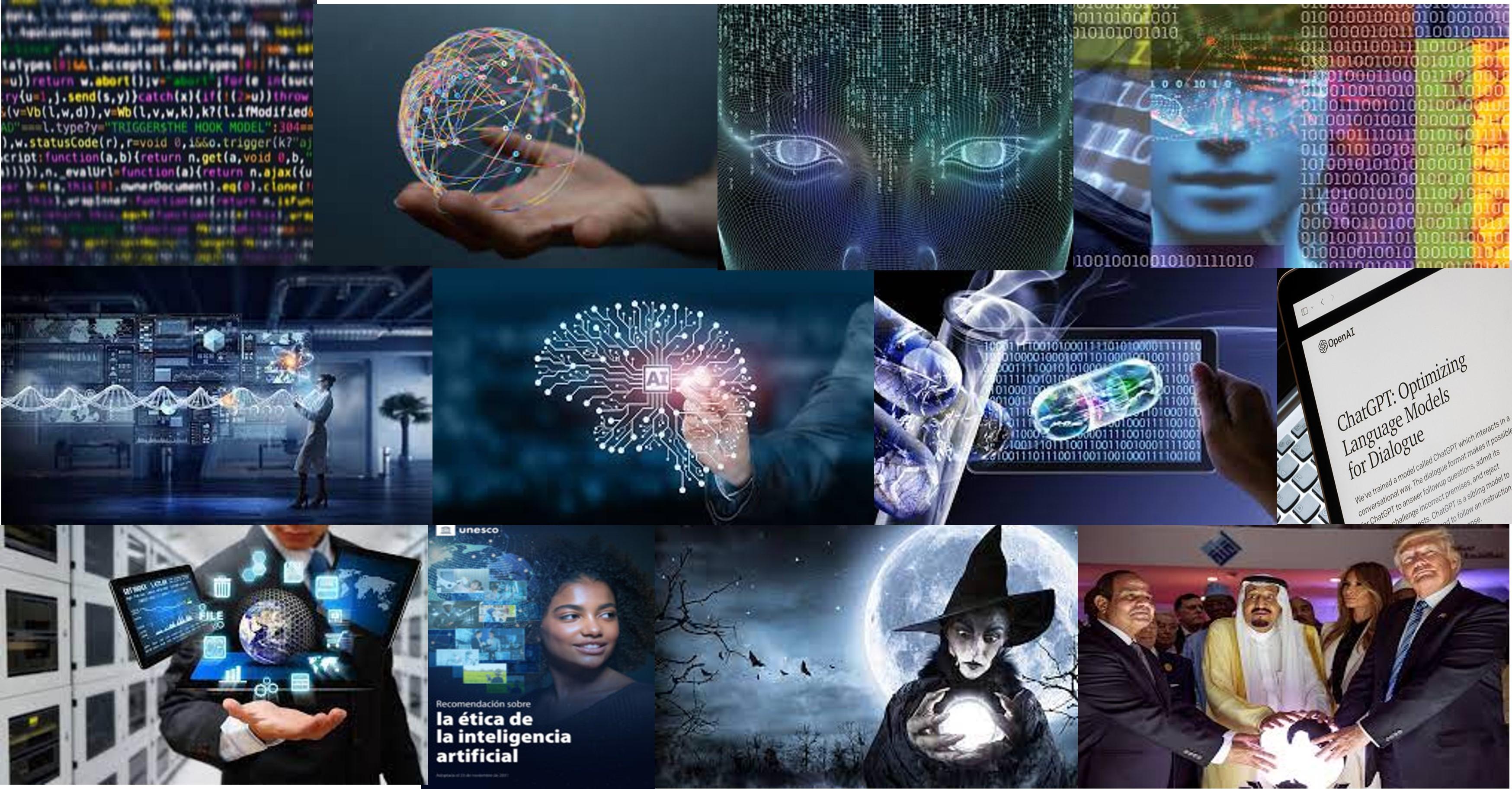
Why Engineers Should Partner with AI—Not Replace Their Judgment
If you ask me, engineering has always been about smart problem-solving—juggling constraints, making trade-offs, and making the best call when things are uncertain. AI can accelerate parts of this by sifting through options or automating some grunt work. But—and I can’t stress this enough—it can’t (and shouldn’t) make the final call for you.
The engineers I respect most treat AI as a teammate. Not a boss, not an intern—an actual partner. They use AI to brainstorm alternatives, catch blind spots, and nudge them toward new perspectives. But when it’s time to make decisions that affect users, systems, or the bottom line? They keep that responsibility for themselves.
Why? Because this isn’t just about avoiding mistakes—it’s about extracting real value from AI. Used well, it doesn’t replace your thinking; it multiplies it.
I’ve seen this play out in collaborative development teams. The groups who actively interact with AI tools—asking questions, pushing back, iterating—consistently ship better code: more readable, more maintainable. Recent research backs this up too: developers who work alongside generative tools outperform those who simply accept whatever output the model gives. Insights on developer augmentation show that the best results come from augmenting developers rather than replacing them.
The ‘Centaur’ approach combines human intuition with machine calculation—letting AI handle repetitive analysis while engineers focus on creative problem-solving and big decisions.
This mindset turns AI from a mysterious black box into a clear collaborator. It keeps technical decisions rooted in your domain expertise, your context, and your ethical compass.
For a deeper dive into how engineers are embracing new roles and mindsets as AI becomes more embedded in workflows, How AI Is Transforming Engineers: Are You a 100,000,000x Engineer? explores what’s changing—and what matters most.
Fluency Isn’t Accuracy: Real Risks of Blind Automation
Here’s something that trips up even seasoned engineers: modern AI sounds incredibly confident and smooth. Large language models like ChatGPT-4 can explain just about anything with polish. But don’t be fooled—sounding smart isn’t the same as being right.
This difference really matters when you’re dealing with edge cases or nuanced specs. Sometimes the answer sounds perfect but totally misses context only an expert would catch.
I’ve struggled with this myself. When I first tried ChatGPT to summarize a design pattern document, I was floored by how readable it was—and then realized it had totally misunderstood the main idea. The fluency masked big errors. If I’d passed those outputs along as-is, my team would have ended up following the wrong patterns entirely.
That wasn’t a one-off. Research comparing AI-written essays to human ones found ChatGPT-4 often scored higher for clarity and structure—but that doesn’t mean the content was actually right or useful for real-world engineering. The quality of generated content study highlights how important it is to look past surface polish and ensure real accuracy. Even when AI-generated code looks clean and maintainable, you still need a human eye on the logic and alignment with project goals.
If you blindly accept AI recommendations, you risk broken builds, confused stakeholders, or wasted time chasing misleading ideas. The discipline here is simple but crucial: treat every suggestion as a hypothesis—a starting point for your own judgment—not a done deal.
To learn how engineers can go beyond surface-level automation and build truly reliable solutions—including lessons learned from failures—see 8 Hard-Won Lessons for Building Reliable Applied AI Agents.
Best Practices: Smarter Ways to Use AI for Engineers
So how do you get the most out of AI without falling into the trap of blind automation? Here’s what I’ve seen work over and over:
Generate Options, Not Decisions
Don’t settle for the first answer AI gives you. Ask for multiple approaches or trade-offs instead. This broadens your perspective and lets you apply your own expertise to choose what fits best.
Supervise Every Output
No matter how polished something looks, treat it as a draft. Before moving code or documentation into production, review it like your name is going on the line—because it is.
Anchor Prompts in Truth
Ground your prompts in real data: your codebase, docs, tickets—whatever’s relevant. Fine-tuning with concrete examples beats clever prompt tricks every time.
Don’t Skip Domain Review
Always bring in subject-matter experts (or just your future self) to audit important outputs. Make sure technical decisions align with architecture, performance requirements, and user needs.
Invest in Feedback Loops
AI isn’t set-and-forget. Keep track of what works and what fails; refine your prompts; notice where the model gets confused or overconfident. Every interaction is a chance to improve both your process and the tool itself.
And here’s a crucial piece that sometimes gets overlooked: safe adoption of large language models (LLMs) demands robust data governance all the way through the project lifecycle. You need clear ownership and traceability on all data sources—see data governance guidance for actionable steps. Companies who take this seriously aren’t just avoiding risk—they’re setting themselves up for real success: organizations with strong governance are three times more likely to hit their goals.
Bottom line? Make these practices part of your routine, and you’ll unlock everything good about AI while keeping its hazards at bay.
For engineers looking to further sharpen their technical decision-making frameworks—especially when weighing automation versus manual approaches—When Manual Beats Automation: Getting Things Done explains why sometimes human-driven solutions win out over pure automation.
Unlocking Leverage: Critical Thinking in the Age of AI
Here’s where the payoff gets big: when you supervise instead of blindly follow AI, you unlock leverage that goes way beyond incremental productivity boosts. You open doors to entirely new ways of solving problems—and building things that wouldn’t have been possible before.
Critical thinking is essential in the age of AI—it protects against automation bias and raises the quality of engineering work across the board.
For engineers navigating new territory or leading teams through complexity and uncertainty, Embracing Uncertainty: The Key to Team Innovation explores how embracing ambiguity and continuous learning leads to stronger solutions and more innovative teams.
AI for engineers isn’t about outsourcing creativity or independent thought—it’s about scaling those very skills across more challenges than ever before. The engineers who thrive will be those who think with machines: asking hard questions, verifying answers, using automation as a springboard for deeper innovation.
So here’s my nudge: master this partnership mindset now. Get curious about what AI can do with you—not for you—and you won’t just keep up as things change; you’ll lead the way.